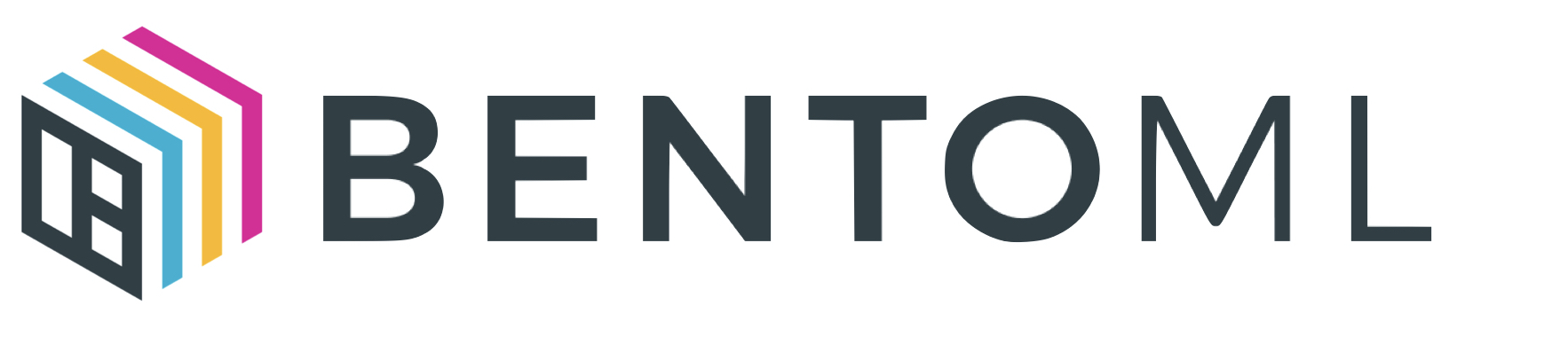
0. Reference
- Naver boostcamp - Product Serving 강의 및 교재
- BentoML Documents https://docs.bentoml.org/en/latest/index.html
- 어쩐지 오늘은 - Machine Learning Serving - BentoML 사용법 https://zzsza.github.io/mlops/2021/04/18/bentoml-basic/
- Line Engineering - MLOps를 위한 BentoML 기능 및 성능 테스트 결과 공유 https://engineering.linecorp.com/ko/blog/mlops-bentoml-1
1. BentoML
1.1 Introduction
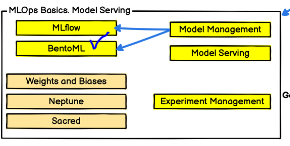
BentoML은 Model을 관리할 수 있는 Serving에 특화된 라이브러리이다.
1.2 BentoML 소개
BentoML이 해결하는 문제
문제 1: Model Serving Infra의 어려움
Serving을 위해 다양한 라이브러리, Artifact, Asset 등 사이즈가 큰 파일을 패키징
Cloud Service에 지속적인 배포를 위한 많은 작업이 필요
BentoML은 CLI로 이 문제의 복잡도를 낮춤(CLI 명령어로 모두 진행 가능하도록)
문제 2: Online Serving의 Monitoring 및 Error Handling
Online Serving으로 API 형태로 생성
Error 처리, Logging을 추가로 구현해야 함
BentoML은 Python Logging Module을 사용해 Access Log, Prediction Log를 기본으로 제공
Config를 수정해 Logging도 커스텀할 수 있고, Prometheus 같은 Metric 수집 서버에 전송할 수 있음
문제 3: Online Serving 퍼포먼스 튜닝의 어려움
BentoML은 Adaptive Micro Batch 방식을 채택해 동시에 많은 요청이 들어와도 높은 처리량을 보여줌
1.3 BentoML 특징
쉬운 사용성
Online / Offline Serving 지원
Tensorflow, PyTorch, Keras, XGBoost 등 Major 프레임워크 지원
Docker, Kubernetes, AWS, Azure 등의 배포 환경 지원 및 가이드 제공
Flask 대비 100배의 처리량
모델 저장소(Yatai) 웹 대시보드 제공
데이터 사이언스와 DevOps 사이의 간격을 이어주며 높은 성능의 Serving이 가능하게 함
2. BentoML 설치 및 사용법
2.1. BentoML 설치
python 3.6 이상 지원
pip install bentoml
2.2 BentoML 사용 Flow
모델 학습 코드 생성
Prediction Service Class 생성
Prediction Service에 모델 저장(Pack)
(Local) Serving
Docker Image Build(컨테이너화)
Serving 배포
모델 학습 코드 생성
Iris Classifier 예제
BentoML Bundle : Prediction Service를 실행할 때 필요한 모든 코드, 구성이 포함된 폴더, 모델 제공을 위한 바이너리
# bento_packer.py # 모델 학습
from sklearn import svm
from sklearn import datasets
clf = svm.SVC(gamma='scale')
iris = datasets.load_iris()
X, y = iris.data, iris.target
clf.fit(X, y) # bento_service.py에서 정의한 IrisClassifier
from bento_service import IrisClassifier # IrisClassifier 인스턴스 생성
iris_classifier_service = IrisClassifier() # Model Artifact를 Pack
iris_classifier_service.pack('model', clf) # Model Serving을 위한 서비스를 Disk에 저장
saved_path = iris_classifier_service.save()
Prediction Service Class 생성
BentoService를 활용해 prediction Services Class 생성
BentoService를 상속하면, 해당 서비스를 Yatai(모델 이미지 레지스트리)에 저장
예측할 때 사용하는 API를 위한 class
@env: 파이썬 패키지, install script 등 서비스에 필요한 의존성을 정의
@artifacts : 서비스에서 사용할 Artifact 정의
- Sklearn
- @artifacts에 사용한 이름을 토대로 self.artifacts.model로 접근
- API에 접근할 때 해당 Method 호출
@api : API 생성
- Input과 Output을 원하는 형태(Dataframe, Tensor, JSON 등)으로 선택할 수 있음
- Doc String으로 Swagger에 들어갈 내용을 추가할 수 있음
# bento_service.py
import pandas as pd
from bentoml import env, artifacts, api, BentoService
from bentoml.adapters import DataframeInput from bentoml.frameworks.sklearn
import SklearnModelArtifact
@env(infer_pip_packages=True) @artifacts([SklearnModelArtifact('model')])
class IrisClassifier(BentoService):
@api(input=DataframeInput(), batch=True)
def predict(self, df: pd.DataFrame):
"""
An inference API named `predict` with Dataframe input adapter, which codifies
how HTTP requests or CSV files are converted to a pandas Dataframe object as the
inference API function input
"""
return self.artifacts.model.predict(df)
Prediction Service 저장(Pack)
service 저장 및 경로 확인
python bento_packer.py
BentoML에 저장된 Prediction Service 확인
bentoml list
저장된 Prediction Service 디렉토리로 이동 후 파일 확인
bentoml.yml에 모델의 메타정보 저장
Dockerfile도 자동으로 생성
Serving
웹 서버로 serving
bentoml serve IrisClassifier:latest
localhost:5000로 접근하면 Swagger UI 확인 가능
로그는 ~/bentoml/logs에 저장
Yatai Service 실행
bentoml yatai-service-start
localhost:3000 에서 Model Repository 확인 가능
Docker Image Build
bentoml containerize IrisClassifier:latest -t iris-classifier
docker 명령어나 FastAPI를 사용하지 않고 웹 서버를 띄우고, 이미지 빌드
Uploaded by
N2T'MLOps' 카테고리의 다른 글
[FastAPI] 경로 매개변수, 쿼리 매개변수 (0) | 2023.06.13 |
---|---|
[mlflow] conda 가상환경에서 mlflow 실행하기 (0) | 2022.12.28 |
[mlflow] mlflow experiments list 이란? mlflow experiments 확인하는 법 (0) | 2022.12.28 |